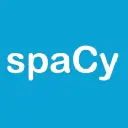
SpaCy
Create powerful NLP applications easily with spaCy.
Top Features
Modular System for Large Language Models
The spacy-llm package integrates Large Language Models (LLMs) into spaCy, providing a modular system that facilitates fast prototyping and prompting. This innovative feature allows users to convert unstructured responses into structured outputs for various NLP tasks, significantly enhancing the output quality without needing training data. This aspect is particularly beneficial for users needing to generate insights quickly and effectively, streamlining the workflow and improving productivity.
Comprehensive Configuration System
spaCy v3.0 introduces a detailed configuration system that allows users to define every aspect of their training runs without hidden defaults. Users can easily rerun experiments and track modifications across different iterations. This transparency not only promotes better understanding and control over the NLP training processes but also enhances reproducibility, which is vital for research and development activities.
Efficient Annotation with Prodigy
Prodigy serves as an exceptional annotation tool that empowers data scientists to undertake their own annotation tasks, significantly speeding up model training and evaluation. Its efficiency in handling entity recognition, intent detection, and even image classification allows teams to iterate rapidly on their models. This unique feature minimizes reliance on dedicated annotators and enhances engagement by making the process more accessible to data scientists.
Pricing
Created For
Data Scientists
Machine Learning Engineers
AI Researchers
Software Developers
Content Strategists
Project Managers
Digital Marketers
Pros & Cons
Pros 🤩
Cons 😑
d
d
d
d
df
df
Pros
spaCy is a powerful and efficient library for Natural Language Processing (NLP), which meets the needs of users looking for speed and ease of use. Its simple installation and productive API make it accessible for beginners and experienced developers alike. The ability to handle large-scale information extraction means it is ideal for processing extensive datasets, fulfilling requirements for performance. The integration of Large Language Models (LLMs) and customizable components allows users to adapt spaCy to various projects, enhancing flexibility. With tools like Prodigy, users can self-annotate data, speeding up model training and ensuring iterative development. Additionally, the project system in spaCy v3.0 helps users keep track of training configurations, simplifying the process of managing experiments.
Cons
Despite its advantages, spaCy has some limitations that could impact user satisfaction. While it provides a well-structured API, users may find its features complex when performing more advanced NLP tasks that require deep customization. Additionally, spaCy might not be as effective for smaller projects where the overhead of installation and setup could outweigh the benefits. Users also need sufficient background in Python to leverage the library fully, which can be a barrier for those with less technical expertise. The focus on large-scale tasks may mean that performance on niche or highly specialized NLP tasks is less optimal. Lastly, while the ecosystem is vast, the reliance on external plugins can sometimes lead to compatibility issues.
Overview
SpaCy is a powerful and user-friendly Natural Language Processing (NLP) tool designed for efficient and scalable applications. It features a modular system that integrates Large Language Models (LLMs), enhancing output quality for various NLP tasks without the need for extensive training data. The comprehensive configuration system in spaCy v3.0 allows users to easily customize and track their training processes, fostering reproducibility in research and development. Prodigy, an annotation tool integrated with spaCy, accelerates data preparation by enabling data scientists to annotate tasks themselves, thus streamlining model training and evaluation. While spaCy excels in large-scale data processing, users may face challenges with real-time processing and a learning curve for those new to programming or NLP.